Suhas Lohit
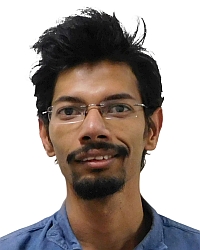
- Phone: 617-621-7569
- Email:
-
Position:
Research / Technical Staff
Principal Research Scientist -
Education:
Ph.D., Arizona State University, 2019 -
Research Areas:
External Links:
Suhas' Quick Links
-
Biography
Before coming to MERL, Suhas worked as an intern at MERL (summer 2018), SRI International (summer 2017) and Nvidia (summer 2016). His research interests include computer vision, computational imaging and deep learning. Recently, his research focus has been on creating hybrid model- and data-driven neural architectures for various applications in imaging and vision. He won the Best Paper Award at the CVPR workshop on Computational Cameras and Displays in 2015 and the University Graduate Fellowship at ASU for 2015-16.
-
Recent News & Events
-
NEWS MERL Papers and Workshops at CVPR 2024 Date: June 17, 2024 - June 21, 2024
Where: Seattle, WA
MERL Contacts: Petros T. Boufounos; Moitreya Chatterjee; Anoop Cherian; Michael J. Jones; Toshiaki Koike-Akino; Jonathan Le Roux; Suhas Lohit; Tim K. Marks; Pedro Miraldo; Jing Liu; Kuan-Chuan Peng; Pu (Perry) Wang; Ye Wang; Matthew Brand
Research Areas: Artificial Intelligence, Computational Sensing, Computer Vision, Machine Learning, Speech & AudioBrief- MERL researchers are presenting 5 conference papers, 3 workshop papers, and are co-organizing two workshops at the CVPR 2024 conference, which will be held in Seattle, June 17-21. CVPR is one of the most prestigious and competitive international conferences in computer vision. Details of MERL contributions are provided below.
CVPR Conference Papers:
1. "TI2V-Zero: Zero-Shot Image Conditioning for Text-to-Video Diffusion Models" by H. Ni, B. Egger, S. Lohit, A. Cherian, Y. Wang, T. Koike-Akino, S. X. Huang, and T. K. Marks
This work enables a pretrained text-to-video (T2V) diffusion model to be additionally conditioned on an input image (first video frame), yielding a text+image to video (TI2V) model. Other than using the pretrained T2V model, our method requires no ("zero") training or fine-tuning. The paper uses a "repeat-and-slide" method and diffusion resampling to synthesize videos from a given starting image and text describing the video content.
Paper: https://www.merl.com/publications/TR2024-059
Project page: https://merl.com/research/highlights/TI2V-Zero
2. "Long-Tailed Anomaly Detection with Learnable Class Names" by C.-H. Ho, K.-C. Peng, and N. Vasconcelos
This work aims to identify defects across various classes without relying on hard-coded class names. We introduce the concept of long-tailed anomaly detection, addressing challenges like class imbalance and dataset variability. Our proposed method combines reconstruction and semantic modules, learning pseudo-class names and utilizing a variational autoencoder for feature synthesis to improve performance in long-tailed datasets, outperforming existing methods in experiments.
Paper: https://www.merl.com/publications/TR2024-040
3. "Gear-NeRF: Free-Viewpoint Rendering and Tracking with Motion-aware Spatio-Temporal Sampling" by X. Liu, Y-W. Tai, C-T. Tang, P. Miraldo, S. Lohit, and M. Chatterjee
This work presents a new strategy for rendering dynamic scenes from novel viewpoints. Our approach is based on stratifying the scene into regions based on the extent of motion of the region, which is automatically determined. Regions with higher motion are permitted a denser spatio-temporal sampling strategy for more faithful rendering of the scene. Additionally, to the best of our knowledge, ours is the first work to enable tracking of objects in the scene from novel views - based on the preferences of a user, provided by a click.
Paper: https://www.merl.com/publications/TR2024-042
4. "SIRA: Scalable Inter-frame Relation and Association for Radar Perception" by R. Yataka, P. Wang, P. T. Boufounos, and R. Takahashi
Overcoming the limitations on radar feature extraction such as low spatial resolution, multipath reflection, and motion blurs, this paper proposes SIRA (Scalable Inter-frame Relation and Association) for scalable radar perception with two designs: 1) extended temporal relation, generalizing the existing temporal relation layer from two frames to multiple inter-frames with temporally regrouped window attention for scalability; and 2) motion consistency track with a pseudo-tracklet generated from observational data for better object association.
Paper: https://www.merl.com/publications/TR2024-041
5. "RILA: Reflective and Imaginative Language Agent for Zero-Shot Semantic Audio-Visual Navigation" by Z. Yang, J. Liu, P. Chen, A. Cherian, T. K. Marks, J. L. Roux, and C. Gan
We leverage Large Language Models (LLM) for zero-shot semantic audio visual navigation. Specifically, by employing multi-modal models to process sensory data, we instruct an LLM-based planner to actively explore the environment by adaptively evaluating and dismissing inaccurate perceptual descriptions.
Paper: https://www.merl.com/publications/TR2024-043
CVPR Workshop Papers:
1. "CoLa-SDF: Controllable Latent StyleSDF for Disentangled 3D Face Generation" by R. Dey, B. Egger, V. Boddeti, Y. Wang, and T. K. Marks
This paper proposes a new method for generating 3D faces and rendering them to images by combining the controllability of nonlinear 3DMMs with the high fidelity of implicit 3D GANs. Inspired by StyleSDF, our model uses a similar architecture but enforces the latent space to match the interpretable and physical parameters of the nonlinear 3D morphable model MOST-GAN.
Paper: https://www.merl.com/publications/TR2024-045
2. “Tracklet-based Explainable Video Anomaly Localization” by A. Singh, M. J. Jones, and E. Learned-Miller
This paper describes a new method for localizing anomalous activity in video of a scene given sample videos of normal activity from the same scene. The method is based on detecting and tracking objects in the scene and estimating high-level attributes of the objects such as their location, size, short-term trajectory and object class. These high-level attributes can then be used to detect unusual activity as well as to provide a human-understandable explanation for what is unusual about the activity.
Paper: https://www.merl.com/publications/TR2024-057
MERL co-organized workshops:
1. "Multimodal Algorithmic Reasoning Workshop" by A. Cherian, K-C. Peng, S. Lohit, M. Chatterjee, H. Zhou, K. Smith, T. K. Marks, J. Mathissen, and J. Tenenbaum
Workshop link: https://marworkshop.github.io/cvpr24/index.html
2. "The 5th Workshop on Fair, Data-Efficient, and Trusted Computer Vision" by K-C. Peng, et al.
Workshop link: https://fadetrcv.github.io/2024/
3. "SuperLoRA: Parameter-Efficient Unified Adaptation for Large Vision Models" by X. Chen, J. Liu, Y. Wang, P. Wang, M. Brand, G. Wang, and T. Koike-Akino
This paper proposes a generalized framework called SuperLoRA that unifies and extends different variants of low-rank adaptation (LoRA). Introducing new options with grouping, folding, shuffling, projection, and tensor decomposition, SuperLoRA offers high flexibility and demonstrates superior performance up to 10-fold gain in parameter efficiency for transfer learning tasks.
Paper: https://www.merl.com/publications/TR2024-062
- MERL researchers are presenting 5 conference papers, 3 workshop papers, and are co-organizing two workshops at the CVPR 2024 conference, which will be held in Seattle, June 17-21. CVPR is one of the most prestigious and competitive international conferences in computer vision. Details of MERL contributions are provided below.
-
TALK [MERL Seminar Series 2024] Melanie Mitchell presents talk titled "The Debate Over 'Understanding' in AI's Large Language Models" Date & Time: Tuesday, February 13, 2024; 1:00 PM
Speaker: Melanie Mitchell, Santa Fe Institute
MERL Host: Suhas Lohit
Research Areas: Artificial Intelligence, Computer Vision, Machine Learning, Human-Computer InteractionAbstractI will survey a current, heated debate in the AI research community on whether large pre-trained language models can be said to "understand" language -- and the physical and social situations language encodes -- in any important sense. I will describe arguments that have been made for and against such understanding, and, more generally, will discuss what methods can be used to fairly evaluate understanding and intelligence in AI systems. I will conclude with key questions for the broader sciences of intelligence that have arisen in light of these discussions.
See All News & Events for Suhas -
-
Awards
-
AWARD Best Paper - Honorable Mention Award at WACV 2021 Date: January 6, 2021
Awarded to: Rushil Anirudh, Suhas Lohit, Pavan Turaga
MERL Contact: Suhas Lohit
Research Areas: Computational Sensing, Computer Vision, Machine LearningBrief- A team of researchers from Mitsubishi Electric Research Laboratories (MERL), Lawrence Livermore National Laboratory (LLNL) and Arizona State University (ASU) received the Best Paper Honorable Mention Award at WACV 2021 for their paper "Generative Patch Priors for Practical Compressive Image Recovery".
The paper proposes a novel model of natural images as a composition of small patches which are obtained from a deep generative network. This is unlike prior approaches where the networks attempt to model image-level distributions and are unable to generalize outside training distributions. The key idea in this paper is that learning patch-level statistics is far easier. As the authors demonstrate, this model can then be used to efficiently solve challenging inverse problems in imaging such as compressive image recovery and inpainting even from very few measurements for diverse natural scenes.
- A team of researchers from Mitsubishi Electric Research Laboratories (MERL), Lawrence Livermore National Laboratory (LLNL) and Arizona State University (ASU) received the Best Paper Honorable Mention Award at WACV 2021 for their paper "Generative Patch Priors for Practical Compressive Image Recovery".
-
-
Research Highlights
-
MERL Publications
- "Equivariant Spatio-Temporal Self-Supervision for LiDAR Object Detection", European Conference on Computer Vision (ECCV), September 2024.BibTeX TR2024-130 PDF Video Presentation
- @inproceedings{Hegde2024sep,
- author = {{Hegde, Deepti and Lohit, Suhas and Peng, Kuan-Chuan and Jones, Michael J. and Patel, Vishal M.}},
- title = {Equivariant Spatio-Temporal Self-Supervision for LiDAR Object Detection},
- booktitle = {European Conference on Computer Vision (ECCV)},
- year = 2024,
- month = sep,
- url = {https://www.merl.com/publications/TR2024-130}
- }
, - "Evaluating Large Vision-and-Language Models on Children's Mathematical Olympiads", arXiv, June 2024.BibTeX arXiv
- @article{Cherian2024jun,
- author = {Cherian, Anoop and Peng, Kuan-Chuan and Lohit, Suhas and Matthiesen, Joanna and Smith, Kevin and Tenenbaum, Joshua B.}},
- title = {Evaluating Large Vision-and-Language Models on Children's Mathematical Olympiads},
- journal = {arXiv},
- year = 2024,
- month = jun,
- url = {https://arxiv.org/abs/2406.15736}
- }
, - "TI2V-Zero: Zero-Shot Image Conditioning for Text-to-Video Diffusion Models", IEEE Conference on Computer Vision and Pattern Recognition (CVPR), June 2024, pp. 9015-9025.BibTeX TR2024-059 PDF Video Software Presentation
- @inproceedings{Ni2024jun,
- author = {Ni, Haomiao and Egger, Bernhard and Lohit, Suhas and Cherian, Anoop and Wang, Ye and Koike-Akino, Toshiaki and Huang, Sharon X. and Marks, Tim K.},
- title = {TI2V-Zero: Zero-Shot Image Conditioning for Text-to-Video Diffusion Models},
- booktitle = {IEEE Conference on Computer Vision and Pattern Recognition (CVPR)},
- year = 2024,
- pages = {9015--9025},
- month = jun,
- url = {https://www.merl.com/publications/TR2024-059}
- }
, - "Gear-NeRF: Free-Viewpoint Rendering and Tracking with Motion-aware Spatio-Temporal Sampling", IEEE Conference on Computer Vision and Pattern Recognition (CVPR), May 2024, pp. 19667-19679.BibTeX TR2024-042 PDF Videos Software
- @inproceedings{Liu2024may,
- author = {Liu, Xinhang and Tai, Yu-wing and Tang, Chi-Keung and Miraldo, Pedro and Lohit, Suhas and Chatterjee, Moitreya},
- title = {Gear-NeRF: Free-Viewpoint Rendering and Tracking with Motion-aware Spatio-Temporal Sampling},
- booktitle = {IEEE Conference on Computer Vision and Pattern Recognition (CVPR)},
- year = 2024,
- pages = {19667--19679},
- month = may,
- publisher = {IEEE},
- url = {https://www.merl.com/publications/TR2024-042}
- }
, - "Multimodal 3D Object Detection on Unseen Domains", arXiv, April 2024. ,
- "Equivariant Spatio-Temporal Self-Supervision for LiDAR Object Detection", European Conference on Computer Vision (ECCV), September 2024.
-
Other Publications
- "Temporal Transformer Networks: Joint Learning of Invariant and Discriminative Time Warping", Proceedings of the IEEE Conference on Computer Vision and Pattern Recognition, 2019, pp. 12426-12435.BibTeX
- @Inproceedings{lohit2019temporal,
- author = {Lohit, Suhas and Wang, Qiao and Turaga, Pavan},
- title = {Temporal Transformer Networks: Joint Learning of Invariant and Discriminative Time Warping},
- booktitle = {Proceedings of the IEEE Conference on Computer Vision and Pattern Recognition},
- year = 2019,
- pages = {12426--12435}
- }
, - "Convolutional neural networks for noniterative reconstruction of compressively sensed images", IEEE Transactions on Computational Imaging, Vol. 4, No. 3, pp. 326-340, 2018.BibTeX
- @Article{lohit2018convolutional,
- author = {Lohit, Suhas and Kulkarni, Kuldeep and Kerviche, Ronan and Turaga, Pavan and Ashok, Amit},
- title = {Convolutional neural networks for noniterative reconstruction of compressively sensed images},
- journal = {IEEE Transactions on Computational Imaging},
- year = 2018,
- volume = 4,
- number = 3,
- pages = {326--340},
- publisher = {IEEE}
- }
, - "Predicting Dynamical Evolution of Human Activities from a Single Image", Proceedings of the IEEE Conference on Computer Vision and Pattern Recognition Workshops, 2018, pp. 383-392.BibTeX
- @Inproceedings{lohit2018predicting,
- author = {Lohit, Suhas and Bansal, Ankan and Shroff, Nitesh and Pillai, Jaishanker and Turaga, Pavan and Chellappa, Rama},
- title = {Predicting Dynamical Evolution of Human Activities from a Single Image},
- booktitle = {Proceedings of the IEEE Conference on Computer Vision and Pattern Recognition Workshops},
- year = 2018,
- pages = {383--392}
- }
, - "Learning invariant Riemannian geometric representations using deep nets", Proceedings of the IEEE International Conference on Computer Vision Workshops, 2017, pp. 1329-1338.BibTeX
- @Inproceedings{lohit2017learning,
- author = {Lohit, Suhas and Turaga, Pavan},
- title = {Learning invariant Riemannian geometric representations using deep nets},
- booktitle = {Proceedings of the IEEE International Conference on Computer Vision Workshops},
- year = 2017,
- pages = {1329--1338}
- }
, - "Reconnet: Non-iterative reconstruction of images from compressively sensed measurements", Proceedings of the IEEE Conference on Computer Vision and Pattern Recognition, 2016, pp. 449-458.BibTeX
- @Inproceedings{kulkarni2016reconnet,
- author = {Kulkarni, Kuldeep and Lohit, Suhas and Turaga, Pavan and Kerviche, Ronan and Ashok, Amit},
- title = {Reconnet: Non-iterative reconstruction of images from compressively sensed measurements},
- booktitle = {Proceedings of the IEEE Conference on Computer Vision and Pattern Recognition},
- year = 2016,
- pages = {449--458}
- }
, - "Direct inference on compressive measurements using convolutional neural networks", 2016 IEEE International Conference on Image Processing (ICIP), 2016, pp. 1913-1917.BibTeX
- @Inproceedings{lohit2016direct,
- author = {Lohit, Suhas and Kulkarni, Kuldeep and Turaga, Pavan},
- title = {Direct inference on compressive measurements using convolutional neural networks},
- booktitle = {2016 IEEE International Conference on Image Processing (ICIP)},
- year = 2016,
- pages = {1913--1917},
- organization = {IEEE}
- }
, - "A statistical estimation framework for energy expenditure of physical activities from a wrist-worn accelerometer", 2016 38th Annual International Conference of the IEEE Engineering in Medicine and Biology Society (EMBC), 2016, pp. 2631-2635.BibTeX
- @Inproceedings{wang2016statistical,
- author = {Wang, Qiao and Lohit, Suhas and Toledo, Meynard John and Buman, Matthew P and Turaga, Pavan},
- title = {A statistical estimation framework for energy expenditure of physical activities from a wrist-worn accelerometer},
- booktitle = {2016 38th Annual International Conference of the IEEE Engineering in Medicine and Biology Society (EMBC)},
- year = 2016,
- pages = {2631--2635},
- organization = {IEEE}
- }
, - "Reconstruction-free inference on compressive measurements", Proceedings of the IEEE Conference on Computer Vision and Pattern Recognition Workshops, 2015, pp. 16-24.BibTeX
- @Inproceedings{lohit2015reconstruction,
- author = {Lohit, Suhas and Kulkarni, Kuldeep and Turaga, Pavan and Wang, Jian and Sankaranarayanan, Aswin C},
- title = {Reconstruction-free inference on compressive measurements},
- booktitle = {Proceedings of the IEEE Conference on Computer Vision and Pattern Recognition Workshops},
- year = 2015,
- pages = {16--24}
- }
,
- "Temporal Transformer Networks: Joint Learning of Invariant and Discriminative Time Warping", Proceedings of the IEEE Conference on Computer Vision and Pattern Recognition, 2019, pp. 12426-12435.
-
Software & Data Downloads
-
Videos
-
MERL Issued Patents
-
Title: "Systems and Methods for Multi-Spectral Image Fusion Using Unrolled Projected Gradient Descent and Convolutinoal Neural Network"
Inventors: Liu, Dehong; Lohit, Suhas; Mansour, Hassan; Boufounos, Petros T.
Patent No.: 10,891,527
Issue Date: Jan 12, 2021
-
Title: "Systems and Methods for Multi-Spectral Image Fusion Using Unrolled Projected Gradient Descent and Convolutinoal Neural Network"