Jing Liu
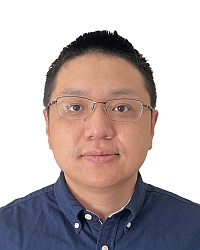
- Phone: 617-621-7584
- Email:
-
Position:
Research / Technical Staff
Visiting Research Scientist -
Education:
Ph.D., University of California, San Diego, 2019 -
Research Areas:
Jing's Quick Links
-
Biography
Before joining MERL, Jing was an Illinois Future Faculty fellow at the Computer Science department of the University of Illinois, Urbana Champaign (UIUC). Prior to that, he was a Postdoctoral Research Associate at the Coordinated Science Lab of UIUC. His research interests include Trustworthy AI, Distributed Learning and Inference, Robust and Efficient Internet-of-Things (IoT), and green AI.
-
Recent News & Events
-
TALK [MERL Seminar Series 2024] Sanmi Koyejo presents talk titled Are Emergent Abilities of Large Language Models a Mirage? Date & Time: Wednesday, March 20, 2024; 1:00 PM
Speaker: Sanmi Koyejo, Stanford University
MERL Host: Jing Liu
Research Areas: Artificial Intelligence, Machine LearningAbstractRecent work claims that large language models display emergent abilities, abilities not present in smaller-scale models that are present in larger-scale models. What makes emergent abilities intriguing is two-fold: their sharpness, transitioning seemingly instantaneously from not present to present, and their unpredictability, appearing at seemingly unforeseeable model scales. Here, we present an alternative explanation for emergent abilities: that for a particular task and model family, when analyzing fixed model outputs, emergent abilities appear due to the researcher's choice of metric rather than due to fundamental changes in model behavior with scale. Specifically, nonlinear or discontinuous metrics produce apparent emergent abilities, whereas linear or continuous metrics produce smooth, continuous predictable changes in model performance. We present our alternative explanation in a simple mathematical model. Via the presented analyses, we provide evidence that alleged emergent abilities evaporate with different metrics or with better statistics, and may not be a fundamental property of scaling AI models.
-
NEWS MERL researchers presenting workshop papers at NeurIPS 2022 Date: December 2, 2022 - December 8, 2022
MERL Contacts: Matthew Brand; Toshiaki Koike-Akino; Jing Liu; Saviz Mowlavi; Kieran Parsons; Ye Wang
Research Areas: Artificial Intelligence, Control, Dynamical Systems, Machine Learning, Signal ProcessingBrief- In addition to 5 papers in recent news (https://www.merl.com/news/news-20221129-1450), MERL researchers presented 2 papers at the NeurIPS Conference Workshop, which was held Dec. 2-8. NeurIPS is one of the most prestigious and competitive international conferences in machine learning.
- “Optimal control of PDEs using physics-informed neural networks” by Saviz Mowlavi and Saleh Nabi
Physics-informed neural networks (PINNs) have recently become a popular method for solving forward and inverse problems governed by partial differential equations (PDEs). By incorporating the residual of the PDE into the loss function of a neural network-based surrogate model for the unknown state, PINNs can seamlessly blend measurement data with physical constraints. Here, we extend this framework to PDE-constrained optimal control problems, for which the governing PDE is fully known and the goal is to find a control variable that minimizes a desired cost objective. We validate the performance of the PINN framework by comparing it to state-of-the-art adjoint-based optimization, which performs gradient descent on the discretized control variable while satisfying the discretized PDE.
- “Learning with noisy labels using low-dimensional model trajectory” by Vasu Singla, Shuchin Aeron, Toshiaki Koike-Akino, Matthew E. Brand, Kieran Parsons, Ye Wang
Noisy annotations in real-world datasets pose a challenge for training deep neural networks (DNNs), detrimentally impacting generalization performance as incorrect labels may be memorized. In this work, we probe the observations that early stopping and low-dimensional subspace learning can help address this issue. First, we show that a prior method is sensitive to the early stopping hyper-parameter. Second, we investigate the effectiveness of PCA, for approximating the optimization trajectory under noisy label information. We propose to estimate the low-rank subspace through robust and structured variants of PCA, namely Robust PCA, and Sparse PCA. We find that the subspace estimated through these variants can be less sensitive to early stopping, and can outperform PCA to achieve better test error when trained on noisy labels.
- In addition, new MERL researcher, Jing Liu, also presented a paper entitled “CoPur: Certifiably Robust Collaborative Inference via Feature Purification" based on his previous work before joining MERL. His paper was elected as a spotlight paper to be highlighted in lightening talks and featured paper panel.
- In addition to 5 papers in recent news (https://www.merl.com/news/news-20221129-1450), MERL researchers presented 2 papers at the NeurIPS Conference Workshop, which was held Dec. 2-8. NeurIPS is one of the most prestigious and competitive international conferences in machine learning.
-
-
Research Highlights
-
MERL Publications
- "SuperLoRA: Parameter-Efficient Unified Adaptation of Multi-Layer Attention Modules", arXiv, March 2024.BibTeX arXiv
- @article{Chen2024mar,
- author = {Chen, Xiangyu and Liu, Jing and Wang, Ye and Wang, Pu and Brand, Matthew and Wang, Guanghui and Koike-Akino, Toshiaki},
- title = {SuperLoRA: Parameter-Efficient Unified Adaptation of Multi-Layer Attention Modules},
- journal = {arXiv},
- year = 2024,
- month = mar,
- url = {https://arxiv.org/abs/2403.11887}
- }
, - "Why Does Differential Privacy with Large ε Defend Against Practical Membership Inference Attacks?", AAAI Workshop on Privacy-Preserving Artificial Intelligence, February 2024.BibTeX TR2024-009 PDF
- @inproceedings{Lowy2024feb2,
- author = {Lowy, Andrew and Li, Zhuohang and Liu, Jing and Koike-Akino, Toshiaki and Parsons, Kieran and Wang, Ye},
- title = {Why Does Differential Privacy with Large ε Defend Against Practical Membership Inference Attacks?},
- booktitle = {AAAI Workshop on Privacy-Preserving Artificial Intelligence},
- year = 2024,
- month = feb,
- url = {https://www.merl.com/publications/TR2024-009}
- }
, - "Exploring User-level Gradient Inversion with a Diffusion Prior", International Workshop on Federated Learning in the Age of Foundation Models in Conjunction with NeurIPS, December 2023.BibTeX TR2023-149 PDF
- @inproceedings{Li2023dec,
- author = {Li, Zhuohang and Lowy, Andrew and Liu, Jing and Koike-Akino, Toshiaki and Malin, Bradley and Parsons, Kieran and Wang, Ye},
- title = {Exploring User-level Gradient Inversion with a Diffusion Prior},
- booktitle = {International Workshop on Federated Learning in the Age of Foundation Models in Conjunction with NeurIPS},
- year = 2023,
- month = dec,
- url = {https://www.merl.com/publications/TR2023-149}
- }
, - "LoDA: Low-Dimensional Adaptation of Large Language Models", Advances in Neural Information Processing Systems (NeurIPS) workshop, December 2023.BibTeX TR2023-150 PDF
- @inproceedings{Liu2023dec,
- author = {Liu, Jing and Koike-Akino, Toshiaki and Wang, Pu and Brand, Matthew and Wang, Ye and Parsons, Kieran},
- title = {LoDA: Low-Dimensional Adaptation of Large Language Models},
- booktitle = {Advances in Neural Information Processing Systems (NeurIPS) workshop},
- year = 2023,
- month = dec,
- url = {https://www.merl.com/publications/TR2023-150}
- }
, - "Stabilizing Subject Transfer in EEG Classification with Divergence Estimation", arXiv, October 2023.BibTeX arXiv
- @article{Smedemark-Margulies2023oct,
- author = {Smedemark-Margulies, Niklas and Wang, Ye and Koike-Akino, Toshiaki and Liu, Jing and Parsons, Kieran and Bicer, Yunus and Erdogmus, Deniz},
- title = {Stabilizing Subject Transfer in EEG Classification with Divergence Estimation},
- journal = {arXiv},
- year = 2023,
- month = oct,
- url = {https://arxiv.org/abs/2310.08762}
- }
,
- "SuperLoRA: Parameter-Efficient Unified Adaptation of Multi-Layer Attention Modules", arXiv, March 2024.
-
Other Publications
- "Robust mean estimation in high dimensions: An outlier fraction agnostic and efficient algorithm", 2022 IEEE International Symposium on Information Theory (ISIT), 2022, pp. 1115-1120.BibTeX
- @Inproceedings{deshmukh2022robust,
- author = {Deshmukh, Aditya and Liu, Jing and Veeravalli, Venugopal V},
- title = {Robust mean estimation in high dimensions: An outlier fraction agnostic and efficient algorithm},
- booktitle = {2022 IEEE International Symposium on Information Theory (ISIT)},
- year = 2022,
- pages = {1115--1120},
- organization = {IEEE}
- }
, - "CoPur: Certifiably Robust Collaborative Inference via Feature Purification", Advances in Neural Information Processing Systems, 2022.BibTeX
- @Inproceedings{liu2022copur,
- author = {Liu, Jing and Xie, Chulin and Koyejo, Oluwasanmi O and Li, Bo},
- title = {CoPur: Certifiably Robust Collaborative Inference via Feature Purification},
- booktitle = {Advances in Neural Information Processing Systems},
- year = 2022
- }
, - "Rvfr: Robust vertical federated learning via feature subspace recovery", NeurIPS Workshop New Frontiers in Federated Learning: Privacy, Fairness, Robustness, Personalization and Data Ownership, 2021.BibTeX
- @Inproceedings{liu2021rvfr,
- author = {Liu, Jing and Xie, Chulin and Kenthapadi, Krishnaram and Koyejo, Sanmi and Li, Bo},
- title = {Rvfr: Robust vertical federated learning via feature subspace recovery},
- booktitle = {NeurIPS Workshop New Frontiers in Federated Learning: Privacy, Fairness, Robustness, Personalization and Data Ownership},
- year = 2021
- }
, - "Information flow optimization in inference networks", ICASSP 2020-2020 IEEE International Conference on Acoustics, Speech and Signal Processing (ICASSP), 2020, pp. 8289-8293.BibTeX
- @Inproceedings{deshmukh2020information,
- author = {Deshmukh, Aditya and Liu, Jing and Veeravalli, Venugopal V and Verma, Gunjan},
- title = {Information flow optimization in inference networks},
- booktitle = {ICASSP 2020-2020 IEEE International Conference on Acoustics, Speech and Signal Processing (ICASSP)},
- year = 2020,
- pages = {8289--8293},
- organization = {IEEE}
- }
, - "Sparse Bayesian learning for robust PCA: Algorithms and analyses", IEEE Transactions on Signal Processing, Vol. 67, No. 22, pp. 5837-5849, 2019.BibTeX
- @Article{liu2019sparse,
- author = {Liu, Jing and Rao, Bhaskar D},
- title = {Sparse Bayesian learning for robust PCA: Algorithms and analyses},
- journal = {IEEE Transactions on Signal Processing},
- year = 2019,
- volume = 67,
- number = 22,
- pages = {5837--5849},
- publisher = {IEEE}
- }
, - "Robust PCA via ℓ0-ℓ1 Regularization", IEEE Transactions on Signal Processing, Vol. 67, No. 2, pp. 535-549, 2018.BibTeX
- @Article{liu2018robust,
- author = {Liu, Jing and Rao, Bhaskar D},
- title = {Robust PCA via ℓ0-ℓ1 Regularization},
- journal = {IEEE Transactions on Signal Processing},
- year = 2018,
- volume = 67,
- number = 2,
- pages = {535--549},
- publisher = {IEEE}
- }
, - "Robust Linear Regression via ℓ0 Regularization", IEEE Transactions on Signal Processing, Vol. 66, No. 3, pp. 698-713, 2017.BibTeX
- @Article{liu2017robust,
- author = {Liu, Jing and Cosman, Pamela C and Rao, Bhaskar D},
- title = {Robust Linear Regression via ℓ0 Regularization},
- journal = {IEEE Transactions on Signal Processing},
- year = 2017,
- volume = 66,
- number = 3,
- pages = {698--713},
- publisher = {IEEE}
- }
,
- "Robust mean estimation in high dimensions: An outlier fraction agnostic and efficient algorithm", 2022 IEEE International Symposium on Information Theory (ISIT), 2022, pp. 1115-1120.