Saviz Mowlavi
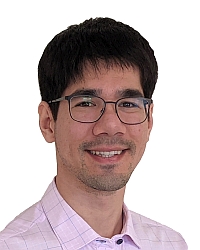
- Phone: 617-621-7588
- Email:
-
Position:
Research / Technical Staff
Research Scientist -
Education:
Ph.D., Massachusetts Institute of Technology, 2022 -
Research Areas:
Saviz's Quick Links
-
Biography
Saviz's research is motivated by the integration of machine learning and physical laws for solving high-dimensional inverse problems in the realm of fluid mechanics, solid mechanics, heat transfer and beyond. During his PhD, he developed computational methods for various physical problems such as detecting hidden voids or inclusions in elastic bodies, identifying coherent structures in ensembles of moving particles, or controlling unstable fluid flows.
-
Recent News & Events
-
NEWS Saviz Mowlavi gave an invited talk at North Carolina State University Date: April 12, 2024
MERL Contact: Saviz Mowlavi
Research Areas: Control, Dynamical Systems, Machine Learning, OptimizationBrief- Saviz Mowlavi was invited to present remotely at the Computational and Applied Mathematics seminar series in the Department of Mathematics at North Carolina State University.
The talk, entitled "Model-based and data-driven prediction and control of spatio-temporal systems", described the use of temporal smoothness to regularize the training of fast surrogate models for PDEs, user-friendly methods for PDE-constrained optimization, and efficient strategies for learning feedback controllers for PDEs.
- Saviz Mowlavi was invited to present remotely at the Computational and Applied Mathematics seminar series in the Department of Mathematics at North Carolina State University.
-
TALK [MERL Seminar Series 2023] Prof. Shaowu Pan presents talk titled Neural Implicit Flow Date & Time: Wednesday, March 1, 2023; 1:00 PM
Speaker: Shaowu Pan, Rensselaer Polytechnic Institute
MERL Host: Saviz Mowlavi
Research Areas: Computational Sensing, Data Analytics, Machine LearningAbstractHigh-dimensional spatio-temporal dynamics can often be encoded in a low-dimensional subspace. Engineering applications for modeling, characterization, design, and control of such large-scale systems often rely on dimensionality reduction to make solutions computationally tractable in real-time. Common existing paradigms for dimensionality reduction include linear methods, such as the singular value decomposition (SVD), and nonlinear methods, such as variants of convolutional autoencoders (CAE). However, these encoding techniques lack the ability to efficiently represent the complexity associated with spatio-temporal data, which often requires variable geometry, non-uniform grid resolution, adaptive meshing, and/or parametric dependencies. To resolve these practical engineering challenges, we propose a general framework called Neural Implicit Flow (NIF) that enables a mesh-agnostic, low-rank representation of large-scale, parametric, spatial-temporal data. NIF consists of two modified multilayer perceptrons (MLPs): (i) ShapeNet, which isolates and represents the spatial complexity, and (ii) ParameterNet, which accounts for any other input complexity, including parametric dependencies, time, and sensor measurements. We demonstrate the utility of NIF for parametric surrogate modeling, enabling the interpretable representation and compression of complex spatio-temporal dynamics, efficient many-spatial-query tasks, and improved generalization performance for sparse reconstruction.
See All News & Events for Saviz -
-
Internships with Saviz
-
ST0105: Internship - Surrogate Modeling for Sound Propagation
MERL is seeking a motivated and qualified individual to work on fast surrogate models for sound emission and propagation from complex vibrating structures, with applications in HVAC noise reduction. The ideal candidate will be a PhD student in engineering or related fields with a solid background in frequency-domain acoustic modeling and numerical techniques for partial differential equations (PDEs). Preferred skills include knowledge of the boundary element method (BEM), data-driven modeling, and physics-informed machine learning. Publication of the results obtained during the internship is expected. The duration is expected to be at least 3 months with a flexible start date.
-
-
MERL Publications
- "Reinforcement Learning-Based Estimation for Spatio-Temporal Systems", Nature Scientific Reports, DOI: 10.1038/s41598-024-72055-1, Vol. 14, pp. 22464, October 2024.BibTeX TR2024-134 PDF
- @article{Mowlavi2024oct,
- author = {Mowlavi, Saviz and Benosman, Mouhacine},
- title = {{Reinforcement Learning-Based Estimation for Spatio-Temporal Systems}},
- journal = {Nature Scientific Reports},
- year = 2024,
- volume = 14,
- pages = 22464,
- month = oct,
- doi = {10.1038/s41598-024-72055-1},
- url = {https://www.merl.com/publications/TR2024-134}
- }
, - "Controlgym: Large-Scale Control Environments for Benchmarking Reinforcement Learning Algorithms", Learning for Dynamics & Control Conference (L4DC), July 2024, pp. 181-196.BibTeX TR2024-098 PDF
- @inproceedings{Zhang2024jul2,
- author = {Zhang, Xiangyuan and Mao, Weichao and Mowlavi, Saviz and Benosman, Mouhacine and Basar, Tamer},
- title = {{Controlgym: Large-Scale Control Environments for Benchmarking Reinforcement Learning Algorithms}},
- booktitle = {Learning for Dynamics \& Control Conference (L4DC)},
- year = 2024,
- pages = {181--196},
- month = jul,
- publisher = {PMLR},
- url = {https://www.merl.com/publications/TR2024-098}
- }
, - "OptiState: State Estimation of Legged Robots using Gated Networks with Transformer-based Vision and Kalman Filtering", IEEE International Conference on Robotics and Automation (ICRA), May 2024.BibTeX TR2024-054 PDF
- @inproceedings{Schperberg2024may,
- author = {Schperberg, Alexander and Tanaka, Yusuke and Mowlavi, Saviz and Xu, Feng and Balaji, Bharathan and Hong, Dennis},
- title = {{OptiState: State Estimation of Legged Robots using Gated Networks with Transformer-based Vision and Kalman Filtering}},
- booktitle = {IEEE International Conference on Robotics and Automation (ICRA)},
- year = 2024,
- month = may,
- publisher = {IEEE},
- url = {https://www.merl.com/publications/TR2024-054}
- }
, - "Policy Optimization for PDE Control with a Warm Start", arXiv, March 2024. ,
- "Smooth and Sparse Latent Dynamics in Operator Learning with Jerk Regularization", arXiv, February 2024. ,
- "Reinforcement Learning-Based Estimation for Spatio-Temporal Systems", Nature Scientific Reports, DOI: 10.1038/s41598-024-72055-1, Vol. 14, pp. 22464, October 2024.
-
Other Publications
- "Topology optimization with physics-informed neural networks: application to noninvasive detection of hidden geometries", arXiv preprint arXiv:2303.09280, 2023.BibTeX
- @Article{mowlavi2023topology,
- author = {Mowlavi, Saviz and Kamrin, Ken},
- title = {Topology optimization with physics-informed neural networks: application to noninvasive detection of hidden geometries},
- journal = {arXiv preprint arXiv:2303.09280},
- year = 2023
- }
, - "Detecting Lagrangian coherent structures from sparse and noisy trajectory data", Journal of Fluid Mechanics, Vol. 948, pp. A4, 2022.BibTeX
- @Article{mowlavi2022detecting,
- author = {Mowlavi, Saviz and Serra, Mattia and Maiorino, Enrico and Mahadevan, L},
- title = {Detecting Lagrangian coherent structures from sparse and noisy trajectory data},
- journal = {Journal of Fluid Mechanics},
- year = 2022,
- volume = 948,
- pages = {A4},
- publisher = {Cambridge University Press}
- }
, - "Contact model for elastically anisotropic bodies and efficient implementation into the discrete element method", Granular Matter, Vol. 23, No. 2, pp. 1-29, 2021.BibTeX
- @Article{mowlavi2021contact,
- author = {Mowlavi, Saviz and Kamrin, Ken},
- title = {Contact model for elastically anisotropic bodies and efficient implementation into the discrete element method},
- journal = {Granular Matter},
- year = 2021,
- volume = 23,
- number = 2,
- pages = {1--29},
- publisher = {Springer}
- }
, - "Interplay between hysteresis and nonlocality during onset and arrest of flow in granular materials", Soft Matter, Vol. 17, No. 31, pp. 7359-7375, 2021.BibTeX
- @Article{mowlavi2021interplay,
- author = {Mowlavi, Saviz and Kamrin, Ken},
- title = {Interplay between hysteresis and nonlocality during onset and arrest of flow in granular materials},
- journal = {Soft Matter},
- year = 2021,
- volume = 17,
- number = 31,
- pages = {7359--7375},
- publisher = {Royal Society of Chemistry}
- }
, - "Reduced model for capillary breakup with thermal gradients: Predictions and computational validation", Physics of Fluids, Vol. 33, No. 12, pp. 122003, 2021.BibTeX
- @Article{shukla2021reduced,
- author = {Shukla, Isha and Wang, Fan and Mowlavi, Saviz and Guyomard, Amy and Liang, Xiangdong and Johnson, Steven G and Nave, J-C},
- title = {Reduced model for capillary breakup with thermal gradients: Predictions and computational validation},
- journal = {Physics of Fluids},
- year = 2021,
- volume = 33,
- number = 12,
- pages = 122003,
- publisher = {AIP Publishing LLC}
- }
, - "Control of linear instabilities by dynamically consistent order reduction on optimally time-dependent modes", Nonlinear Dynamics, Vol. 95, No. 4, pp. 2745-2764, 2019.BibTeX
- @Article{blanchard2019control,
- author = {Blanchard, Antoine and Mowlavi, Saviz and Sapsis, Themistoklis P},
- title = {Control of linear instabilities by dynamically consistent order reduction on optimally time-dependent modes},
- journal = {Nonlinear Dynamics},
- year = 2019,
- volume = 95,
- number = 4,
- pages = {2745--2764},
- publisher = {Springer}
- }
, - "Particle size selection in capillary instability of locally heated coaxial fiber", Physical Review Fluids, Vol. 4, No. 6, pp. 064003, 2019.BibTeX
- @Article{mowlavi2019particle,
- author = {Mowlavi, Saviz and Shukla, Isha and Brun, P-T and Gallaire, Fran{\c{c}}ois},
- title = {Particle size selection in capillary instability of locally heated coaxial fiber},
- journal = {Physical Review Fluids},
- year = 2019,
- volume = 4,
- number = 6,
- pages = 064003,
- publisher = {APS}
- }
, - "Absolute/convective secondary instabilities and the role of confinement in free shear layers", Physical Review Fluids, Vol. 3, No. 5, pp. 053901, 2018.BibTeX
- @Article{arratia2018absolute,
- author = {Arratia, Crist{\'o}bal and Mowlavi, Saviz and Gallaire, Fran{\c{c}}ois},
- title = {Absolute/convective secondary instabilities and the role of confinement in free shear layers},
- journal = {Physical Review Fluids},
- year = 2018,
- volume = 3,
- number = 5,
- pages = 053901,
- publisher = {APS}
- }
, - "Model order reduction for stochastic dynamical systems with continuous symmetries", SIAM Journal on Scientific Computing, Vol. 40, No. 3, pp. A1669-A1695, 2018.BibTeX
- @Article{mowlavi2018model,
- author = {Mowlavi, Saviz and Sapsis, Themistoklis P},
- title = {Model order reduction for stochastic dynamical systems with continuous symmetries},
- journal = {SIAM Journal on Scientific Computing},
- year = 2018,
- volume = 40,
- number = 3,
- pages = {A1669--A1695},
- publisher = {SIAM}
- }
, - "Spatio-temporal stability of the Kármán vortex street and the effect of confinement", Journal of Fluid Mechanics, Vol. 795, pp. 187-209, 2016.BibTeX
- @Article{mowlavi2016spatio,
- author = {Mowlavi, Saviz and Arratia, Crist{\'o}bal and Gallaire, Fran{\c{c}}ois},
- title = {Spatio-temporal stability of the K{\'a}rm{\'a}n vortex street and the effect of confinement},
- journal = {Journal of Fluid Mechanics},
- year = 2016,
- volume = 795,
- pages = {187--209},
- publisher = {Cambridge University Press}
- }
, - "In vivo observations and in vitro experiments on the oral phase of swallowing of Newtonian and shear-thinning liquids", Journal of Biomechanics, Vol. 49, No. 16, pp. 3788-3795, 2016.BibTeX
- @Article{mowlavi2016vivo,
- author = {Mowlavi, S and Engmann, J and Burbidge, A and Lloyd, R and Hayoun, P and Le Reverend, B and Ramaioli, M},
- title = {In vivo observations and in vitro experiments on the oral phase of swallowing of Newtonian and shear-thinning liquids},
- journal = {Journal of Biomechanics},
- year = 2016,
- volume = 49,
- number = 16,
- pages = {3788--3795},
- publisher = {Elsevier}
- }
, - "A model experiment to understand the oral phase of swallowing of Newtonian liquids", Journal of biomechanics, Vol. 48, No. 14, pp. 3922-3928, 2015.BibTeX
- @Article{hayoun2015model,
- author = {Hayoun, P and Engmann, J and Mowlavi, S and Le Reverend, B and Burbidge, A and Ramaioli, M},
- title = {A model experiment to understand the oral phase of swallowing of Newtonian liquids},
- journal = {Journal of biomechanics},
- year = 2015,
- volume = 48,
- number = 14,
- pages = {3922--3928},
- publisher = {Elsevier}
- }
,
- "Topology optimization with physics-informed neural networks: application to noninvasive detection of hidden geometries", arXiv preprint arXiv:2303.09280, 2023.
-
MERL Issued Patents
-
Title: "Time-varying reinforcement learning for robust adaptive estimator design with application to HVAC flow control"
Inventors: Nabi, Saleh; Benosman, Mouhacine; Mowlavi, Saviz
Patent No.: 12,313,276
Issue Date: May 27, 2025
-
Title: "Time-varying reinforcement learning for robust adaptive estimator design with application to HVAC flow control"