MERL’s Virtual Open House 2024
November 19, 2024
Join us for MERL's virtual open house 2024 on November 19th. Live sessions will be held from 1:00-4:30pm EST, including an overview of recent activities by our research groups, a featured guest speaker and live interaction with our research staff through the Gather platform. Registered attendees will be able to browse our virtual booths at their convenience and connect with our research staff on engagement opportunities, including internship/post-doc openings as well as visiting faculty positions.
Details
- Date: Tuesday, November 19, 2024
- Time: 1:00 - 4:30 PM EST
Live Session Schedule
1:00 - 1:25 EST |
Auditorium A Welcome / Opening Remarks Anthony Vetro |
|||
1:30 - 2:10 EST |
Auditorium A Keynote "Representation-based Learning and Control for Dynamical Systems" Na LI, Harvard University |
|||
Group Introduction & Opportunities | ||||
2:20 - 2:40 EST |
Auditorium A Speech & Audio Jonathan Le Roux |
Auditorium B Computational Sensing Petros T. Boufounos |
Auditorium C Electric Systems Automation Yebin Wang |
Auditorium D Optimization & Intelligent Robotics Arvind Raghunathan |
2:40 - 3:00 EST |
Auditorium A Computer Vision Tim K. Marks |
Auditorium B Connectivity and Information Processing Kieran Parsons |
Auditorium C Controls for Autonomy Stefano Di Cairano |
Auditorium D Multi-Physical Systems Chris R. Laughman |
3:00 - 4:30 EST | Open Interaction on Gather Platform |
Virtual Exhibit Booths
Attendees are invited to visit our virtual booths on Gather platform to learn more about MERL's research activities and internship opportunities. These virtual spaces will provide:
- Materials that provide a more in-depth view of our latest research results
- Links to relevant internship, post-doc, and full-time opportunities
- An opportunity to interact live with MERL researchers
The event will feature 18 virtual booths in the following research areas:
- 3D Computer Vision
- Multimodal Reasoning and Generative AI for Computer Vision
- Visual Analysis
- Optimization Algorithms & Power Systems
- Human-Robot Interaction
- Robotic Manipulation
- Mobile Robotics and Drones
- Autonomy for Spacecraft
- High-dimensional Dynamical Systems
- Computational Sensing
- Multimodal scene understanding and Generative AI
- Acoustic and audio analysis
- Physics informed learning for design/control
- Predictive Maintenance for Electric Systems
- Sustainable and Trustworthy AI
- IoT Communications
- Model-based Design and Control for Multiphysical Systems
- Machine Learning and Estimation for Digital Twins
Featured Guest Speaker
Prof. Na Li, Harvard UniversityRepresentation-based Learning and Control for Dynamical Systems
Abstract: The explosive growth of machine learning and data-driven methodologies have revolutionized numerous fields. Yet, the translation of these successes to the domain of dynamical physical systems remains a significant challenge. Closing the loop from data to actions in these systems faces many difficulties, stemming from the need for sample efficiency and computational feasibility, along with many other requirements such as verifiability, robustness, and safety. In this talk, we bridge this gap by introducing innovative representations to develop nonlinear stochastic control and reinforcement learning methods. Key in the representation is to represent the stochastic, nonlinear dynamics linearly onto a nonlinear feature space. We present a comprehensive framework to develop control and learning strategies which achieve efficiency, safety, robustness, and scalability with provable performance. We also show how the representation could be used to close the sim-to-real gap. Lastly, we will briefly present some concrete real-world applications, discussing how domain knowledge is applied in practice to further close the loop from data to actions.
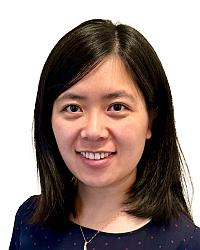
Dr. Na Li is a Winokur Family Professor of Electrical Engineering and Applied Mathematics at Harvard University and a visiting researcher in Mitsubishi Electric Research laboratories (MERL). She received her Bachelor's degree in Mathematics from Zhejiang University in 2007 and Ph.D. degree in Control and Dynamical systems from California Institute of Technology in 2013. She was a postdoctoral associate at the Massachusetts Institute of Technology 2013-2014. She has held a variety of short-term visiting appointments including the Simons Institute for the Theory of Computing, MIT, and Google Brain. Her research lies in the control, learning, and optimization of networked systems, including theory development, algorithm design, and applications to real-world cyber-physical societal system. She has been an associate editor for IEEE Transactions on Automatic Control, Systems & Control Letters, IEEE Control Systems Letters, and served on the organizing committee for a few conferences. She received the NSF career award, AFSOR Young Investigator Award, ONR Young Investigator Award, Donald P. Eckman Award, McDonald Mentoring Award, IFAC Distinguished Lecture, and IFAC Manfred Thoma Medal, along with other awards.
Contact Us
If you are experiencing any issues with registration or accessing the event site, or would like further information about this event, please contact us at
.