TR2002-39
Multidimensional Visualization for Collaborative Filtering Recommender Systems
-
- "Multidimensional Visualization for Collaborative Filtering Recommender Systems", Tech. Rep. TR2002-39, Mitsubishi Electric Research Laboratories, Cambridge, MA, September 2002.BibTeX TR2002-39 PDF
- @techreport{MERL_TR2002-39,
- author = {Frederick J. Igo Jr., Matthew Brand, Kent Wittenburg, David Wong and Shinsuke Azuma},
- title = {Multidimensional Visualization for Collaborative Filtering Recommender Systems},
- institution = {MERL - Mitsubishi Electric Research Laboratories},
- address = {Cambridge, MA 02139},
- number = {TR2002-39},
- month = sep,
- year = 2002,
- url = {https://www.merl.com/publications/TR2002-39/}
- }
,
- "Multidimensional Visualization for Collaborative Filtering Recommender Systems", Tech. Rep. TR2002-39, Mitsubishi Electric Research Laboratories, Cambridge, MA, September 2002.
-
MERL Contacts:
-
Research Areas:
Data Analytics, Human-Computer Interaction
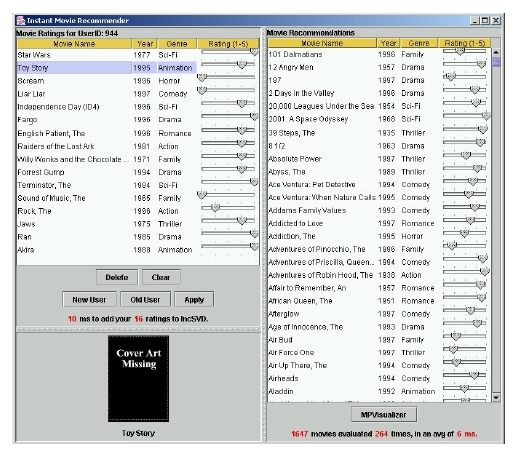
Abstract:
This paper describes a movie recommender system that demonstrates both an incremental SVD prediction algorithm as well as multidimensional visualization of the prediction results. The recommender system makes accurate recommendations in real-time and supports incremental updating, also in real time. In addition the movie recommendation system demonstrates the advantages of multidimensional visualization of the recommender system's results. Recommendations for all movies, along with several attributes of the movies being recommended, are viewed in parallel as bargrams in the multidimensional visualization tool. This tool allows the user to browse the recommendations and filter the results using multiple movie attributes at once. The user can dynamically navigate to a short list of recommendations with only a few clicks. By adding recommendations for other users as additional dimensions we also demonstrate how the system can recommend for groups just as easily as for individuals.